Data centres are the backbone of the digital economy, and they are undergoing a period of rapid transformation. The rise of artificial intelligence (AI) is driving the need for new infrastructure to support the demanding workloads of AI applications.
In a recent roundtable discussion in Hong Kong, a group of leading IT professionals gathered to discuss the challenges and opportunities that surround next-generation data centre infrastructure. Hosted by CXOCIETY with Digital Realty and Telstra International, the roundtable examined how AI was reshaping their infrastructure requirements and creating new challenges around data sovereignty, security, and sustainability.
The power challenge
The most immediate impact of AI on data centre infrastructure is the unprecedented power density requirements of AI workloads.
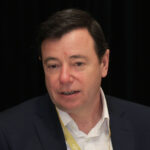
Roddie Samuel, VP of Sales at Digital Realty, emphasised the increasing power demands in data centres, with some AI environments now reaching upwards of 70 kilowatts per cabinet. He added that next-generation technologies like advancements in Nvidia’s GPUs would drive these requirements even higher, prompting urgent infrastructure adaptations and sparking conversations about innovative cooling methods and alternative energy solutions.
“There’s a lot of conversation happening around incorporating alternative energy sources,” said Samuel. “The priorities are shifting. It’s no longer just about having the data centre close to your users — it’s increasingly about being close to the power source.”
Distributed infrastructure takes centre stage
The participants agreed that AI is changing the traditional centralised data centre model into a more distributed approach. This is driven by a host of factors, from latency requirements to regulatory compliance.
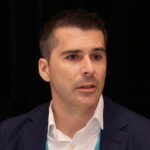
Andrija Condor, Data Centre and Cloud senior sales specialist at Telstra International, highlighted a growing trend: “We see this huge growth of traffic going under subsea cables that we own and operate. And it’s not just coming from the hyperscalers — increasingly, it comes from enterprises. It’s not uncommon for big banks or investment companies to go with high-capacity connectivity across Asia, which was just a couple of years ago all meant for hyperscalers.”
The same power-hungry AI workloads are also generating vast amounts of data. This data needs to be stored, processed, and analysed, driving the need for more efficient and scalable data centre infrastructure.
Condor noted that AI was increasing the demand for more distributed data centre infrastructure. He highlighted the challenges that enterprise customers face, including decisions on where to place their data, how to manage its location, whether to segregate or consolidate it and where to run workloads effectively.
Navigating data sovereignty and compliance in Hong Kong
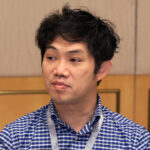
For enterprises in Hong Kong, adhering to strict data sovereignty and regulatory requirements introduces other significant infrastructure challenges. Percy Kong, managing director of the Treasury IT Project Team at ICBC Asia, noted that many organisations face the need to relocate their data centres to meet evolving compliance standards. “Many Chinese banks are required to migrate their data centres into China … It’s not just about finding a technology solution; compliance is a critical factor as well.”
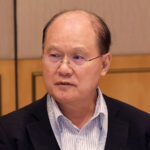
The increasingly clinical regulatory landscape is driving organisations to adopt a more “federated” approach to infrastructure and partnerships. Philip Tam, former CIO-Asia at Veolia, shared how his organisation collaborated with local partners while maintaining oversight and alignment with corporate headquarters to ensure compliance.
Security in the AI era
The impact of AI on security is a growing concern. The delegates noted that many conventional security tools weren’t ready to fight AI. AI is also speeding up malware development, allowing hackers to target specific companies in a matter of hours.
At the same time, AI helps companies improve their security posture. Participants from banking and financial services companies especially appreciated the ability to use AI to enhance security measures and speed up eKYC processes for accounting. They also saw it as helping to tip the balance in detecting and preventing AI attacks themselves.
Balancing cost efficiency and AI investments for long-term ROI
Physical infrastructure isn’t the only challenge. The participants highlighted the importance of being cost-efficient and maximising AI investments to generate long-term returns, particularly in the context of balancing infrastructure needs and the rapid evolution of Generative AI (GenAI) models.
One pressing concern raised was the significant resources required to train these highly complex models, which often become outdated within three to six months. While fine-tuning can enhance performance, the question remains: how much investment is sustainable for technology with such a short lifecycle?
The growing demand for skilled workers to manage next-generation data centres, especially those proficient in AI and data science, presents a challenge due to the ongoing talent shortage. As GenAI models evolve, the need for a highly skilled workforce becomes even more critical.
“There are very few people who can manage these environments,” said Samuel. “This will become even more critical in the near future, which is why a supportive partner ecosystem is essential.” Collaborating with technology vendors helps close skill gaps, optimise costs, and ensure long-term returns on AI investments.
Early days of adoption
Despite the urgency to adapt infrastructure for AI, participants agreed that enterprises were still in the early stages of adoption. They noted that everyone in the market was exploring the potential of GenAI and how it fits with their corporate goals. Many also saw AI as a journey and not just a solution.
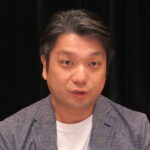
But it’s not just finding the right use cases. Edward Wu, Group IT head of Business Intelligence for Data Governance at WeLab saw a major challenge in bringing internal Proofs of Concept (PoCs) into production, especially when moving from training AI models to inference. “We’ve developed numerous internal use cases already, but the challenge lies in transitioning them into a phase of broader adoption,” he explained.
For some enterprises, the focus is currently on smaller-scale implementations. They want to see how GenAI tools like Microsoft Copilot or Adobe Firefly can help streamline their daily operations and free their teams to do more strategic tasks. However, balancing licensing costs with productivity gains is a struggle.
Navigating an uncertain future
As AI technologies continue to evolve at an accelerating rate, businesses must act swiftly to ensure their systems are scalable and adaptable. Delaying infrastructure upgrades or failing to anticipate future demands could lead to missed opportunities, inefficient operations, and a lag in competitiveness.
The evolving landscape necessitates a proactive approach, integrating flexibility and advanced capabilities to handle the growing complexities of AI models and the data they generate.
As Condor from Telstra observes, “Most of the companies are in the phase of testing Copilot (and other AI models), going through all the pain of how do we comply? How do we keep the regulators happy? How do we make sure that our cyber teams are happy? How do we make sure that data is not leaking?”
Samuel from Digital Realty emphasised the urgency, noting, “There’s so much we don’t know, but it’s moving quickly, and we see it globally. The US is moving much faster than other regions. We need to take risks, or we’ll be left behind — and it’s not just companies, but governments too.” He also stressed that to stay competitive in this rapidly evolving landscape, organisations must invest in AI-ready platforms that support higher power density, cooling, and scalability.
As demand for AI capabilities accelerates, transforming the data centre infrastructure is essential. The challenges of managing AI workloads, ensuring compliance, addressing power needs, and securing data are reshaping the business landscape. Adopting next-generation data centres requires both technical innovation and strategic ecosystem support.
By embracing AI-ready platforms, leveraging partnerships, and investing in skilled talent, organisations can stay ahead, optimising long-term returns. As Samuel from Digital Realty stated, the time to act is now — those who fail to adapt risk being left behind.
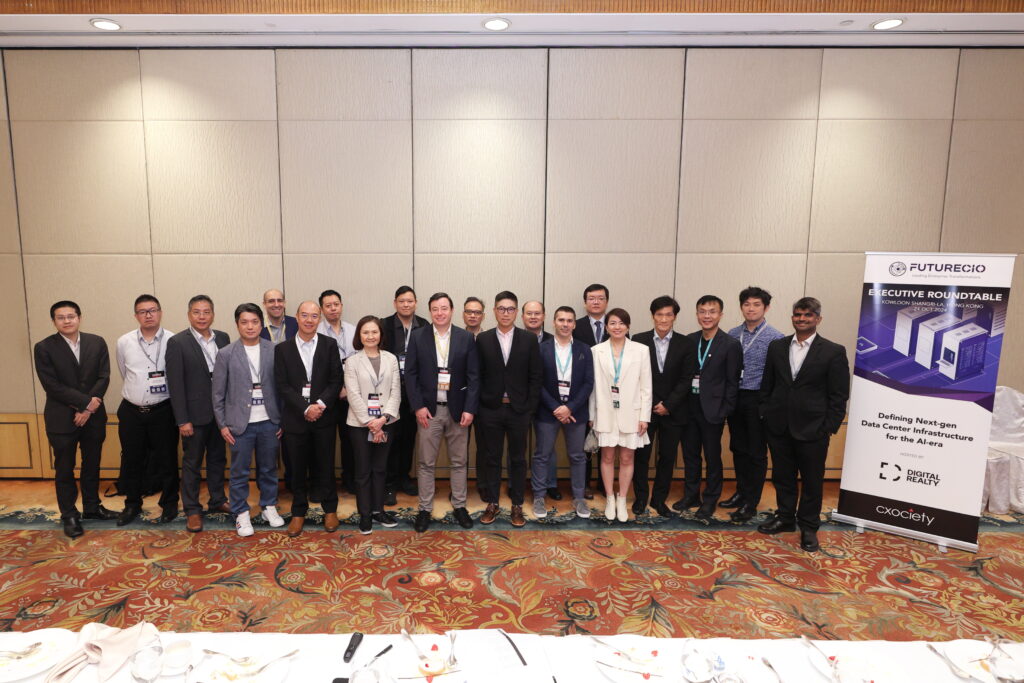
Source: Cxociety