A Gartner survey of 644 organisations (Q4 2023) revealed that data availability remains one of the top five barriers to implementing generative AI (GenAI), specifically, obtaining real-world data and labelling it.
Gartner says that with orders of magnitude less privacy risk than real data, synthetic data can open a range of opportunities to train machine learning (ML) models and analyse data that would not be available if real data were the only option.
Artificially generated and typically created using algorithms, synthetic data can be deployed to validate mathematical models and to train machine learning models.
Tan Ser Yean, CTO of IBM Singapore, explains that synthetic data mimics the properties of the original data, ensuring its similarity to real-world data while eliminating any sensitive or personally identifiable information (PII).
He explains that synthetic data is an important asset as it is information generated on a computer to augment or replace real data to improve AI models, protect sensitive data, and mitigate bias.
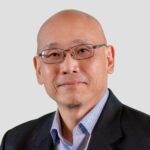
“Synthetic data is typically cheaper to produce, comes automatically labelled, and sidesteps many of the logistical, ethical, and privacy issues that come with training deep learning models on real-world examples.”
Tan Ser Yean
As technology vendors, industry practitioners and regulators develop their understanding of artificial intelligence, their awareness of the risks associated with the technology rises.
Ensuring privacy protection
Asked how synthetic data generation techniques can ensure privacy protection, Bugcrowd CEO Dave Gerry says depersonalisation methods introduce noise to or iteratively anonymise the data, making it tough to trace back to individuals while maintaining the overall data patterns.
“This ideally means that an LLM would not be able to compromise individuals’ privacy with such data sets. Advanced models like Generative Adversarial Networks (GANs) generate synthetic datasets that are useful like the original data but without the risk of privacy leaks,” he goes on to explain.
Synthetic data real-world use cases
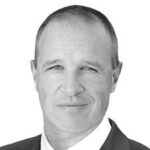
Alexander Linden, VP analyst at Gartner, says organisations can use synthetic data to test a new system where no live data exists or when data is biased. They can also take advantage of synthetic data to supplement small, existing datasets that are currently being ignored. “Alternatively, they choose synthetic data when real data can’t be used, can’t be shared or can’t be moved,” he added.
IBM’s Tan says synthetic data generation is being adopted by organisations in machine learning model development. He says in the finance sector, synthetic data is employed to create financial transactions, including banking, payments, credit cards, and customer profiles. “These datasets are then utilised to build machine learning models for fraud detection, credit risk scoring, and know your customer (KYC) purposes, thereby enhancing the efficiency and personalisation of financial services,” he declared.
In healthcare, he reveals that synthetic data are used to simulate patient profiles, electronic health records (EHR), and electronic medical records (EMR), which are then harnessed to train AI models for precise medicine, medical diagnosis, healthcare resource allocation, and population health management. This enables better healthcare services and outcomes for citizens.
“In each of these industries, synthetic data generation offers several advantages, such as reduced data privacy risks, accelerated model development, and improved model performance,” said Tan. “By adopting this technology, organisations can better protect sensitive information and still enable the development of advanced machine learning models.”
Gartner’s Linden says the breadth of its applicability will make synthetic data a critical accelerator for AI. “Synthetic data makes AI possible where lack of data makes AI unusable due to bias or inability to recognize rare or unprecedented scenarios,” he continued.
Addressing the biases in synthetic data
One of the early revelations around machine learning and artificial intelligence is that there is bias in the content created by the technology. Tan acknowledges that synthetic data can introduce new biases if the generation algorithm is not properly designed.
“A lack of diversity and randomness in synthetic data may misrepresent the real-world scenario, causing the AI model to be biased or overfit,” he begins. “For example, if a synthetic data generation algorithm fails to include age ranges or ethnicities in the generated data, the AI model trained on this data may perpetuate existing biases present in the real-world data.”
To overcome the limitations, Tan suggests that synthetic data generators utilise a wide array of real-world data sources, including structured, semi-structured, and unstructured data, to create artificial data that accurately represents the complexity and diversity of the original data.
“This ensures that the generated synthetic data can be used in a variety of applications and scenarios,” opines Tan. “Organisations should also establish clear data governance practices to ensure that synthetic data is generated and used responsibly, transparently, and ethically.
“This includes establishing policies and procedures for data collection, storage, access, and sharing, as well as ensuring that appropriate security measures are in place to protect the synthetic data,” he further elaborates.
Bugcrowd’s Gerry says it is important to regularly audit the synthetic data through AI bias assessments from experts who can identify and mitigate potential biases in the data generation process and update models as needed to address and correct any new biases that might emerge over time.
Effectiveness of synthetic data
One of the themes of digitalisation is the desire to achieve greater efficiency while keeping costs down. It can be argued that this focus on efficiency has reached the point of being industrialised.
So, how do we validate the effectiveness of synthetic data for ML models?
Tan believes that enterprise-wide adoption would require business leaders and data scientists to have confidence in the quality of the synthetic data output. He suggests they quickly grasp how closely the synthetic data maintains the statistical properties of their existing data model.
“One important metric is “fidelity”, which assesses the quality of the synthetic data in terms of its similarity to real data and the data model. Enterprises should gain insight not only into column distributions but also into the relationships between other columns, both one-to-one (univariate) and one-to-many (multivariate).”
Tan believes it is also valuable to understand the utility of synthetic data in AI model training before sharing it with the appropriate teams. “Essentially, this metric measures the relative predictive accuracy of a machine learning model when trained on real data compared to synthetic data,” he adds.
Fairness is gaining prominence due to potential biases present in enterprise-collected datasets.
“Gaining insight into the extent of this bias can help enterprises recognise and potentially correct it. While not as prevalent in today’s synthetic data solutions and not as critical as privacy, fidelity or utility, understanding the bias in one’s synthetic data will help enterprises make more informed decisions.”
Tan Ser Yean
Protecting synthetic data repositories
The pandemic may have paved the way for the accelerated migration to the cloud but it has also opened the eyes of finance and the board on the financial perils of uncontrolled spending. As CFOs query IT about its unbridled spending on all things cloud, it won’t be long before CIOs will face the same concerns around artificial intelligence.
Tan says companies should consider on-premises deployments when their synthetic data has dependencies on existing sensitive data. “Third-party cloud providers often offer robust built-in security and privacy safeguards. However, sending and storing sensitive PII customer data in such clouds may expose the organisation to potential risks,” he cautioned.
He also suggests that risk, security and compliance leaders implement a mechanism to control their desired level of privacy risk during the synthetic data generation process. “Differential privacy” is one such mechanism, enabling data scientists and risk teams to manage their desired level of privacy (typically within an epsilon range of 1 to 10, with 1 representing the highest privacy).
“This method masks the contribution of any individual, making it impossible to infer specific information about a person, including whether their information was used at all,” he explained.
“Lastly, when differential privacy is not an option, business users should maintain a line of sight into privacy-related metrics – allowing them to comprehend the extent of their privacy exposure,” he concluded.
For his part, Gerry suggests the use of encryption for data both at rest and in transit to guard against unauthorised access. “Homomorphic encryption that allows processing of encrypted data could be used. Strict access controls and authentication mechanisms must be in place to ensure that only authorized personnel can access the synthetic data.
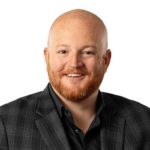
“Regular AI Penetration testing should be conducted to identify and address security weaknesses. It's also important to maintain logs of data access and monitor for any suspicious activities, ensuring that any anomalies can be quickly identified and addressed.”
Dave Gerry
“Finally, applying anonymization techniques adds an extra layer of protection to the identities within synthetic datasets, further safeguarding sensitive information,” he concluded.