The emergence of artificial intelligence (AI) agents has ignited much discussion across industries, including the finance sector, where they are touted to trigger a major shift with their ability to reason and carry out tasks autonomously.
Asked how the use of AI has evolved in finance, BlackLine CTO Jeremy Ung pointed to agentic AI, which he described as the most significant development for the sector over the past year.
It brings together the power of large language models (LLMs) and reasoning to think “like a human”, executing the necessary steps to carry out tasks, Ung said in a video interview with FutureCIO.
The industry had moved from pure data science to machine learning, then to AI and LLM, and now to agentic AI, which combines various processes to generate more human-like output and reasoning, said Ung, who has more than 20 years of experience in software engineering.
It closes a gap, where AI had been able to support tasks such as anomaly detection and -- with the emergence of GenAI -- report summarisation, but lacked the ability to make decisions.
Agentic AI can plug this with its ability to power decision making, driving further workflow automation, which is critical for finance, Ung said. He noted that there still is a lot of potential to automate workflows and have these be agentic.
BlackLine already has been tapping AI, including generative AI (GenAI), and machine learning capabilities across its products, he added. These encompass functions such as document summarisation and record generation.
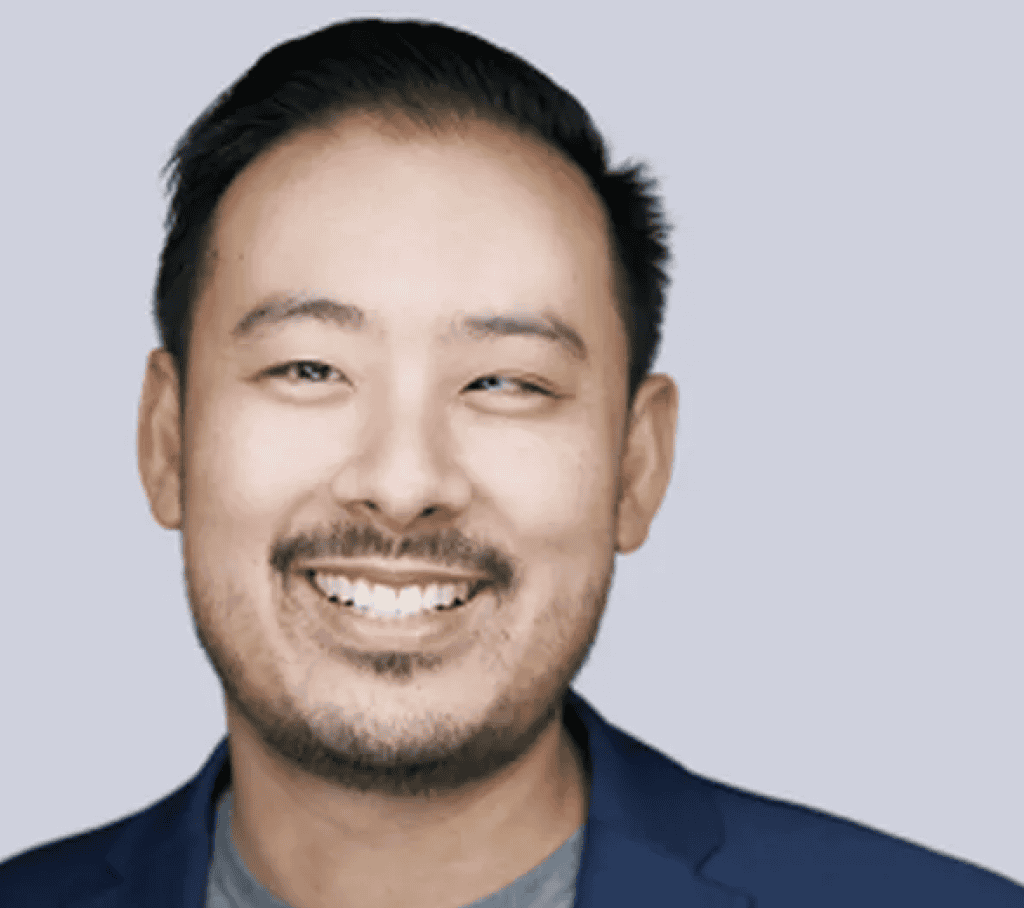
The enterprise software vendor aims to bring autonomous finance to its customers, using AI to automate tasks and augment human judgement, he said.
And it isn’t the only one with such aspirations.
Some 70% of Asia-Pacific organisations anticipate agentic AI to disrupt business models in the next 18 months, according to a March 2025 study from IDC, which polled 300 respondents across the region.
As it is, 5% of respondents believe the technology already has impacted their business.
IDC further noted that agentic AI represented the next frontier of AI, integrating decision making, task execution, and multi-agent collaboration into business workflows.
Specifically for the financial industry, agentic AI will bring about a transformative era, accelerating data processing and improving decision accuracy, noted the World Economic Forum (WEF) in a report last December.
The global body added that, unlike GenAI models that respond to specific human prompts, agentic AI can autonomously perceive, reason, act, and learn without constant human guidance.
The technology can personalise customer interactions and adapt to complex market conditions, pushing the finance sector closer to process autonomy, WEF said. Powered by LLMs, AI agents work collectively using advanced reasoning and planning capabilities to solve multi-step problems, it said.
“Imagine a trading AI agent that analyses market data and autonomously monitors market trends, deciphers trading signals, adjusts strategies, and mitigates risks in real-time,” WEF said.
With AI agents handling repetitive data-intensive processes, financial institutions can better optimise workflows, enhance compliance, and improve decision-making. The agentic AI era will transform how financial services conduct business and interact with customers, WEF added.
“However, it is crucial to recognise the importance and trust when implementing [agentic AI] systems,” said Deepika Giri, IDC Asia-Pacific’s head of research for big data and AI. “The underlying data ecosystem must evolve to support agent-based architectures by enabling dynamic data pipelines that facilitate the seamless flow of multimodal data across systems. The rise of multi-agent system architectures will represent the next major wave in AI adoption.”
AI data accuracy crucial in finance
The finance sector, in particular, is adverse to risk so data accuracy is a top priority with regards to agentic AI, and AI in general, said Ung.
“They’re dealing with numbers, so there’s very little room for error,” he said. “They need to be assured they can trust the [AI-generated] answers.”
He stressed that users should remain in the driver’s seat and should have the ability to accept or reject and refine AI-powered outputs.
This continuous data refinement with real-world use cases also helps build out AI models, including BlackLine’s, and train them to generate better responses, he said. Data is aggregated and anonymised, as well as non-identifiable, to maintain the customer’s privacy, he added.
Organisations are transforming to have data be the underpinning infrastructure, which will allow them to orchestrate workflows, Ung said. This encompasses efforts to move data out of silos and spreadsheets, and bringing them together into data lakes, he said.
It also further underscores the need to empower finance teams with data that is accurate, efficient, and timely, to power their workflows and decisions, he noted.
Organisations, hence, need to know what kind of data they own as well as the quality of the data, so they can determine and mitigate any potential risks, Ung said.
“Not understanding the risks is a pitfall, as is not understanding the opportunities [data brings],” he said. “It’s not [simply] about eliminating spreadsheets, but about having the tools to make these more efficient, more accurate, and more intelligent.”
It also is not a zero-sums game, he added, noting that agentic AI does not need to be the only solution. Likewise, an LLM may not be the best way to forecast finances, he said, where there may be better, smaller AI models to facilitate that.
Furthermore, it takes time for users to trust new technologies, such as agentic AI, he added.
Companies should not lose sight of the critical requirements in any AI initiative, including understanding what AI models are used, how outcomes are achieved, and that these are recorded, Ung said.
If deployed well, though, AI agents could bring potential benefits to the finance industry.
Noting that the sector is the second largest consumer of GenAI after telecom and media, Citibank said in a January 2025 report that this momentum will continue with agentic AI, where use cases span several areas.
These include automating routine tasks of context-aware workflows, real-time risk profiling, tax and retirement planning agents, real-time anti-money laundering compliance, and detection of contextual-based suspicious activities, the bank said.