With a surge in big data, companies face a growing challenge to make their business data-driven. Many have departments that operate in silos with little or almost no unified data strategy.
Seventy per cent of companies do not have a well-articulated data strategy. Over 60% do not manage data as a business asset. While 58.8% compete on analytics, according to a 2021 NewVantage Partners Big Data and AI Executive Survey.
Recently, senior business, technology and operational leaders gathered for a roundtable discussion on “The challenges of Developing an Operational Data Strategy for AI-Ready, Data-Centric Business”, hosted by Cxociety and MicroStrategy in Singapore.
Data and its users
Delegates recognized that raw data has no business value unless it is put to use.
Data is everywhere. It can be of value if you can extract insights out of it and based on that, relate it to your clients and make better business decisions. Data has to have a point of view.
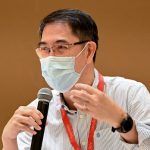
“Each user will have his questions and standpoint. For example, in the motor vehicle industry, somebody will look at it from the safety standpoint, someone else will look at it from the engine standpoint,” said David Tay, chief information officer at Beyonics.
Challenges with mining data
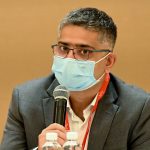
“My issue is around the efficiency part of it. We are dealing with so much data and the challenge is to create the space to generate insights. Data and application processes in large organizations can be complex. By the time you have data that is clean, the amount of time left for generating insights is limited,” said Arjun Jolly, general manager of downstream reporting & analysis at Shell.
He also acknowledged that data strategy must be refreshed regularly. “But any strategy can be as simple and clear as possible but to execute the data strategy through the organization is a challenge. Defining a data strategy itself is not normally difficult. Efficient & effective execution is where the challenge lies.”
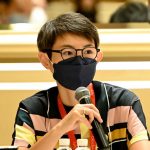
Timeliness can be an issue. “It is not a question of insufficient data. Nowadays we are getting rather too much data. The issue is how to transfer data into real value to the business. We need to identify essential data driving the performance. And we are obligated to the execution of such data-driven strategy,” said Joy Bao, senior manager, profitability analytics group at EPAM Systems.
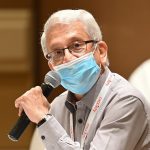
“I prefer answers based on the hypothesis. There is so much data, 80% of it is more like junk or duplicate data. So when you have a data strategy and hypothesis, the data can then be actionable,” added Raju Chellam, chief editor of the AI E&G BoK, an initiative by the Singapore Computer Society and Infocomm Media Development Authority.
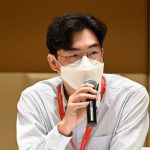
“You have people who have hypotheses and people who have questions, and we have people who own data. Are they the same one? The problem we are seeing today is we have business users who are much more data-savvy or analytics literate, and these people have very good business questions. Unfortunately, the people who own the data are not these business users and they do not have those business questions,” highlighted Chung Kyung-Whu, sales engineering director, APAC of MicroStrategy.
“There is the efficiency issue as well,” he added. “When a user has a business question, it is passed to the IT person. The IT person then will create a pipeline and begins creating the reports. This process will take two weeks if data is already available. When there are follow-up questions, the IT person will run the same process. This resulted in inefficiency as the entire process takes too long,” said Chung.
Silos and execution challenges
Silo data is another problem many participants are trying to overcome because it can affect the value of the data.
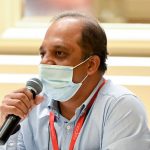
“When we have a data lake setup for silo units or functions, it is extremely challenging to get the right insight out of this data from an overall organizational perspective. Many organizations are struggling with the silo approach,” said Azuhar Mohammed, head of global solution centre expertise & innovation at Sanofi.
“The key question is who is going to take ownership of the data and drive it? Different businesses units will have different expectations when it comes to what information or insight we want to derive out of the data. How can we use data to gain insights or predictions in such a situation?” he added.
“When data is in silos, you will not be able to do analytics because it is still silo analytics and will only be effective for that silo. You will not be able to touch the other parts of the organization, which is a big problem,” added Chellam.
“When we have different IT platforms or solutions with its own data definition and data management techniques within different business functions, the business does not get the real value of it. Therefore, an integrated data approach with clearly defined roles and responsibilities and with right end-to-end data ownership is vital for any organization to drive their digital transformation journey,” said Mohammed.
Elements of a data strategy
According to Chung, people, process, and platform are the key elements to an effective data strategy.
“Data strategy has a few elements. With the platform element, we must think about the data requirement approach,” he continued. He gave the example of an approach at Tesla where the company removes all the data and gathers only what is necessary.
He added that another approach is to gather everything first. But there is a disconnect between the people who ask the question and the people who gather the data.
“Which level of empowerment can we give to the people? How do you empower people to find answers to their questions? Organizations must have a process to support their data strategy,” commented Chung.
The process can also involve ownership of data and governance. “We treat data as an asset. Once you generate data in a company, you need policies and strategies to protect your data. You cannot leave it to the individual to decide whether it is important or unimportant. Data needs to be protected. What you do with it, how you process it and what insights you get out of it is vital,” said Chellam.
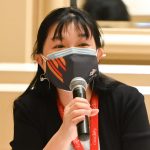
“It is a good time to ask ourselves what kind of data will contribute to that data transformation and how we are monitoring the data. There are various sets of data for different business functions. The classification of the data is also important. The data governance strategy and ownership. Who has that data and where is the accountability? That is very important in the maturity of an organization,” added Jayne Leow, head of enterprise (IT) at SMRT Corporation.
“We have different systems. Systems are important but the governance aspect as we grow will become key in terms of scalability. We have users that can change the system, migrate to the cloud. The thing that stops these projects is the security and accessibility,” she expanded.
Best practices
According to Shell’s Jolly: “One best practice is that we use a common language when it comes to the business. For more than 400 business entities around the world, everyone uses the same chart of accounts, the same structure for management info and we don't have any challenges with a common language which is a significant achievement for our scale of operation.”
He went on to explain that the challenge is the quality of data, and the multiple lenses that businesses need data in an ever-complex world, to ensure the data accuracy at the initial part are critical. We, at the reporting group, are at the end of the data process.
“We spend a lot of time cleaning or making sense of the data whether there is a sale at the retail station or divestment of an asset. The data life cycle is critical. We are focusing on reducing the number of manual entries or manual journals. We all have manual entries, and we work hard to minimise the use of manual entries, every time we kill a manual journal, we are fixing the broken part of the process and making the data life cycle much stronger. We spend a lot of time on that and have seen some success in that area,” he added.
Another best practice is the management of people. “We need people to decide to make the final call. Data will never be the full story and [there are areas] that cannot be quantified and cannot be captured with data. So it is important to have pp to make the decision,” said Beyonics’ Tay.
“Let all those smart devices and applications use it as a channel to deliver the insight that attracts them to execute and implement. That is something we can think about and put the AI into that context,” he suggested.