Forrester principal analyst Mike Gualtieri says there are two types of artificial intelligence (AI). Pure AI is something that is not achievable in present time. Pure AI is the stuff of science fiction movies that is so far removed from reality, it is not five years into the future. Enterprises can use pragmatic AI.
“Pragmatic AI is narrower in scope but can often exceed human intelligence because it is analysing a lot of data. A good example is (IBM) Watson that beat the Jeopardy Champions as it was an AI set to win Jeopardy. But you can’t just snap your fingers and all of a sudden that system becomes a doctor, a lawyer or any other job,” said Gualtieri.
Because pragmatic AI is narrower in scope, it can be used in many enterprise use cases.
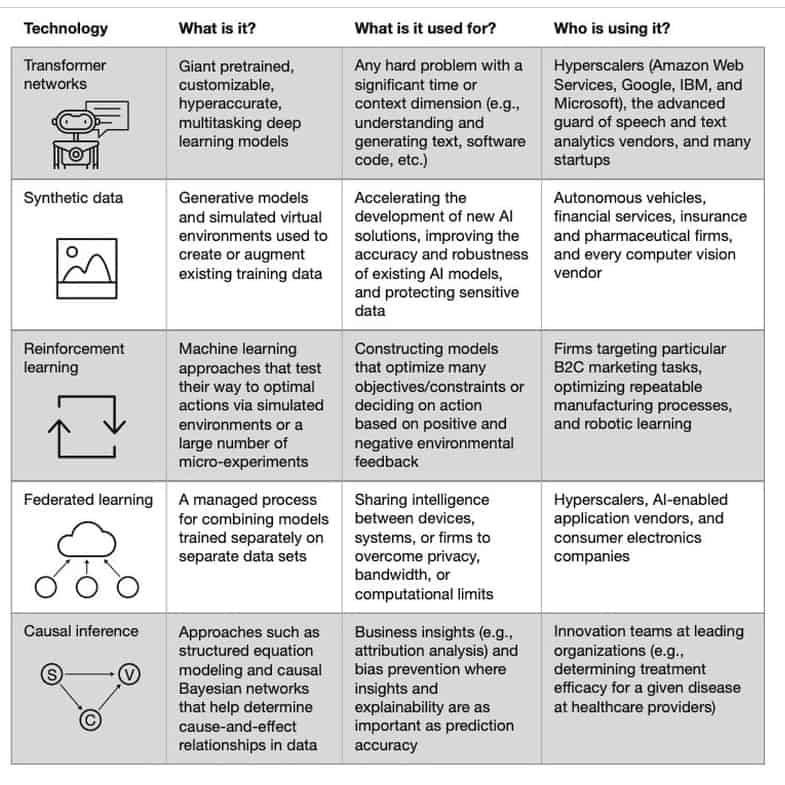
Transformer networks
These are large pretrained, customizable, hyper-accurate, multitasking deep learning models that can be used for any hard problem with a significant time or context dimension (e.g. understanding and generating text, software code, etc.).
Synthetic data
AI models can become expensive because of the requirement for large repositories of data. The solution is generative models and simulated virtual environments that are used to create or augment existing training data. These can be used to accelerate the development of new AI solutions, improving the accuracy and robustness of existing AI models and protecting sensitive data.
Reinforcement learning
Possibly the stuff of science fiction movies, this is a machine that learns as it interacts with its environment at scale. Reinforcement learning is about machine learning approaches that test their way to optimal actions via simulated environments or a large number of micro-experiments that can be used for constructing models that optimize many objectives/constraints or deciding on action based on positive and negative environmental feedback.
Federated learning
A managed process for combining models trained separately on separate data sets that can be used for sharing intelligence between devices, systems, or firms to overcome privacy, bandwidth, or computational limits.
Causal inference
Approaches such as structured equation modelling and causal Bayesian networks that help determine cause-and-effect relationships in data that can be used for business insights and bias prevention where insights and explainability are as important as prediction accuracy.
Forrester claims these advances are already entering commercial products, and forward-looking enterprises need to start preparing if they want to reap their competitive advantages.