Gartner defines a large language model (LLM) as a specialised type of artificial intelligence (AI) that has been trained on vast amounts of text to understand existing content and generate original content.
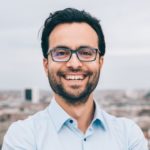
Cem Dilmegani, a principal analyst at AIMultiple, says LLMs are foundation models that utilise deep learning in natural language processing (NLP) and natural language generation (NLG) tasks. Using techniques such as fine-tuning, in-context learning, and zero-/one-/few-shot learning, these models can be adapted for downstream (specific) tasks such s question answering, sentiment analysis, object recognition and following instructions.
Use cases for LLMs
According to IBM’s Institute of Business Value (IBV), AI can enhance customer experience by 70%. In addition, AI can increase productivity in HR by 40% and in application modernisation by 30%.
Nick Gorga, general manager for HPC and AI, Asia Pacific and India at HPE cites other uses cases including include intelligent chatbots or virtual assistants to enhance customer experience, detection of financial fraud and security risks, and real-time analysis of large amounts of unstructured data to inform decision-making and solve business problems.
Dinesh Varadharajan, chief product officer with Kissflow, says enterprises can simplify work for employees by enabling teams to build enterprise-grade apps embedded with LLMs to automate workflows and increase productivity.
Kalyan Madala, chief technology officer with IBM Technology for ASEANZK, believes that it is important to reevaluate prior automation scope based on the new generative AI capabilities. "Many design, composition, and summarisation tasks in processes were not addressed by the prior automation wave, and in some cases, even those areas automated with AI peaked at lower performance levels than are possible today.
Optimising LLM developments and deployment
Because LLMs depend on a repository of data to establish context, Iterate.ai CTO, Brian Sathianathan says real-time LLM applications can take more time to make an inference and may need more compute resources as part of the process.
"It’s crucial to understand which use cases should use LLMs versus “traditional” AI. Another technique is better curating training data—distilling it into a compute-friendly dataset. There’s also quantisation optimisation, reducing compute to 4-8 bits so the LLM runs faster on a cheaper CPU or GPU," he commented.
Following this train of thinking, Gorga believes the first step to establishing an optimal LLM development is for enterprises to identify their specific use cases, followed by determining requirements of resources from computing, and storage to manpower.
"Once the LLM has been deployed – on-premises or in the cloud, enterprises should continually monitor and evaluate its performance and resource utilisation to maintain the quality of outcomes and troubleshoot when needed while optimising operations," he added.
Varadharajan suggests that to move at the speed of technology, CIOs must form internal structures and teams that support speed.
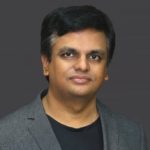
"Siloed teams and networks cause delays and are resource intensive. Encouraging multi-disciplinary teams to consistently innovate drives an enterprise’s progress."
Dinesh Varadharajan
"CIOs should invest in low-code software which increases the accessibility of development across organisations while generative AI increases organisational efficiency, freeing up employees’ time from repetitive tasks. This will foster greater innovation, collaboration, and skills development," he opined.
For his part, Madala says GenAI and LLM investments need to be focused on the highest-impact and most practical use cases—to optimise value for the organisation and to provide models for future priorities.
He suggests establishing performance-based compensation and rewards that align with business goals to maximise generative AI readiness among staff. He also suggests taking an iterative approach to generative AI roll-out that encourages risk-taking and failing fast.
He also suggests holding business, IT, and HR leaders jointly accountable for generative AI outcomes.
"This will help amplify teamwork and underscore the strategic importance of generative AI adoption across the enterprise, benefiting your organisation as a whole. From a purely technology standpoint, take a platform-oriented approach which preserves your strategic optionality of which models to use, and the location to deliver services from. Avoiding lock-in is going to be key," said Madala.
Mitigating the potential risks of deploying LLMs
As with any new emerging technologies, adopting LLMs carries any number of risks from misinformation and disinformation to privacy concerns, to ethical and legal considerations.
Especially for contextually nuanced queries, Gorga says users are usually recommended to verify the information generated to avoid propagating misinformation, as any LLM model is only as reliable as the data it's trained on.
On the privacy front, he cautioned that LLMs can often expose the sensitive or private information of users, from the training data, without their explicit consent or awareness. "Hence, users should be sufficiently careful when dealing with data holding personal information," he added.
For his part, Sathianathan cited hallucinations such as a customer receiving a wrong answer in sensitive circumstances, for example, while making a bank withdrawal.
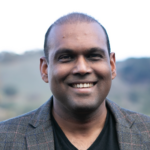
"One mitigating approach is to include guardrails for certain interactions, leveraging traditional machine learning for sensitive cases and LLMs for generic cases. Also, choosing a model with fewer parameters will reduce hallucinations."
Brian Sathianathan
Varadharajan says CIOs must be ready to restructure their strategies time and again as business objectives and goals evolve along with new technologies.
He posits that employee retention is a rising concern, as employees need to be assured that LLMs will help reduce their backlogs instead of increasing their workload.
"New skills are required to keep pace with emerging technologies. A software-defined infrastructure will enable enterprises to maximise investments by integrating software that improves application performance, streamlines management, and safeguards data," he continued.
Accuracy and ethical considerations
As with artificial intelligence, LLMs are vulnerable to producing biased responses. Gorga says enterprises should diversify training data so that LLMs are not trained on biased datasets. He also suggests employing experts in diversity and inclusion to review and audit LLMs to ensure they are not perpetuating bias.
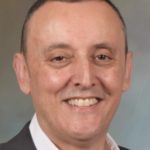
"Businesses also need to establish clear data privacy and security guidelines by implementing data encryption and access controls to protect sensitive data. Lastly, organisations should consider establishing ethics committees to assess the ethical implications as well as providing training for employees to navigate ethical considerations."
Nick Gorga
Varadharajan believes that enterprises need to have visibility of their “digital footprint” of all user activity within their platform, for safeguarding against unauthorised utilisation of data.
"To improve data accuracy, eliminate bias, and ensure compliance with ethical standards, enterprises should curate from broad and representative datasets, and humans should cross-check outputs on a regular basis to find anomalies and fix any inaccuracies," he added.
Eering on caution, Malada posits that Generative AI and AI solutions must be developed carefully and with critical attention paid to the potential risks involved including bias, opacity, hallucination, security, intellectual property rights, and performance and precision management over the lifecycle of the model. Generative AI deployments bring in additional concerns around hate, abuse, and profanity (HAP)—or leak of personally identifiable information (PII).
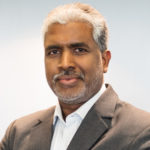
"To address these risks, enterprises need to enhance their governance, risk and compliance (GRC) processes with tools and model validation aligned to principles such as fairness, ethics, accountability and transparency, and ensure performance management is using integrated and automated solutions to ensure speed of execution."
Kalyan Madala
"Finally, AI depends on good data, there is no AI without information architecture (IA); all information governance best practices still apply," he concluded.