The success of the iPhone (2007) can be attributed to one characteristic – its intuitive interface that meant the average user can use the device out of the box without reading the manual, and without being a geek.
An added feature of the iPhone that came a few years later (2011) extended the device’s usability further – and even spawned a movie and memes – was Siri, a virtual assistant that owes its innovation to natural language processing (NLP) and speech recognition.
Over the years, advances in machine learning, algorithm, and some might argue – artificial intelligence – continues to impact the development of such technologies.
Lux Research considers natural language processing (NLP) and topic modelling the AI tools of choice, for innovation leaders as they seek to extract from value and leverage data for maximum impact.
In Lux Research’s whitepaper, Improving the Front End of Innovation with Artificial Intelligence and Machine Learning, the researcher claims NLP can improve processes including technology landscaping, competitive analysis, and weak signal detection.
NLP enables rapid analysis of huge volumes of text, which is where most of the data driving innovation lives.
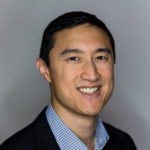
“When utilised effectively, machine learning can quickly mine data to produce actionable insights, significantly decreasing the time it takes for a comprehensive analysis to be performed. An analysis that would have previously taken weeks can now be reduced to days,” said Kevin See, Ph.D., vp of Digital Products for Lux Research.
The speed conferred through NLP is enabled by the comprehensiveness of topic modelling, which extracts important concepts from text while eliminating the human assumption and bias associated with it.
“Previously, an investigation was hindered by either the limited knowledge or bias of the primary investigator, both of which are mitigated when using machine learning. A beneficial technology or idea is less likely to be missed due to an error in human judgement,” explained See.
Lux Research suggests offers some points as organisations define their AI and machine learning strategy including whether you’ll buy or build your technology, what data sources you’ll use, and how you’ll leverage experts to define and interpret the data.
It’s also important to adapt a culture of acceptance of these tools so that valued human resources see them as an asset to their skills rather than competition.
“The confidence and speed AI and machine learning bring to the decision-making process is enabling innovation to happen at a more rapid pace than ever before, but don’t think this means humans are no longer needed,” See added.
People are still necessary to define the starting points of an analysis, label topics, and extract insights from the data collected.
“It’s clear that a collaboration between humans and machines can generate better results, benefiting all involved,” See continued.