A chatbot is an artificial intelligence program that chats with you. The first chatbot, Eliza, was developed by MIT professor Joseph Weizenbaum in the 1960s. Six decades later, we have ChatGPT, Google Gemini, and Microsoft Copilot, joining Alexa and Siri.
What are AI agents?
While chatbots use pattern matching and natural language processing to interpret user inputs and choose the correct responses from pre-programmed options, AI agents analyse data, make decisions, and integrate with various tools and environments, acting as "supercharged assistants".
AI agents leverage large language models (LLMs) to execute various practical tasks, such as filling out forms, researching information, and summarising data. They are LLMs that are given agency, usually function calling in a RAG (retrieval-augmented generation) setting, to make high-level decisions about how to perform tasks in an environment.
According to David Irecki, chief technology officer for Asia Pacific and Japan at Boomi, "AI agents are software components designed with a specific purpose and personality, enabling them to perform tasks with or without human intervention." He adds that "these agents use AI-driven reasoning to independently determine how to accomplish tasks and take appropriate actions," automating tasks "ranging from simple to complex, without requiring constant human input."
Irecki also notes the rise of "emerging multi-agent models", which "allow AI agents to work together seamlessly to handle complex tasks." He gives the example of a travel booking agent orchestrating other AI agents to book flights, hotels, and car rentals, delivering a complete travel package.
Hype vs reality
While the potential of AI agents is immense, it's essential to acknowledge the current limitations. Gary Marcus, professor of Psychology and Neural Science at New York University and a noted AI researcher, suggests that while AI agents will eventually become commonplace and valuable, the current offerings are essentially demos that may not work reliably. "All that we will have this year are demos. And those demos won't work all that reliably," Marcus notes.
Irecki observes, "Based on discussions with both business operations and technology leaders in 2024, there is a fair amount of misalignment around what AI agents truly are." This misalignment contributes to the gap between expectations and actual implementations.
One of the primary challenges is ensuring reliability. Reports indicate that current AI agents can be "extremely brittle" and easily disrupted by real-world complexities. This is partly due to the unresolved issue of hallucinations, where AI systems generate inaccurate or nonsensical information.
The WebArena leaderboard, which benchmarks LLM agents against real-world tasks, shows that even the best-performing models have a success rate of only 45.7%.
Furthermore, Irecki highlights a Gartner report indicating that "only 54% of AI projects transitioned from pilot to production in 2024, and 30% of those in production will be abandoned by 2025." He points out that "training AI models on low-quality data remains a major challenge" hindering successful deployment.
Real-world applications
Despite these challenges, AI agents are already making inroads in various industries, including e-commerce, where they recommend products and help manage inventory; healthcare, where they can assist in diagnosing diseases and creating treatment plans; finance, where they help detect fraud and analyse market trends, and transportation, where they help optimise delivery routes and enable autonomous driving.
"By taking over routine tasks, AI agents allow employees to focus on higher-value work. AI agents help organisations automate repetitive tasks, improving efficiency, speed, and accuracy while enabling 24/7 operations." David Irecki
He adds, "AI agents contribute to personalising services and enhancing customer experience, which can lead to increased business growth and productivity."
A small "big" problem
However, while AI agents are becoming a widespread topic of discussion during break time among those seriously considering the technology, the more significant impediment to integrating it into the workplace is data quality.
According to a report by Boomi titled A Playbook for Crafting AI Strategy, produced in partnership with MIT Technology Review Insights, half of respondents cite data quality as the most limiting data issue in AI deployment. Organisations need a data foundation to adopt AI agents effectively and benefit from increased productivity and cost savings.
Potential risks
As AI agents become more sophisticated, they also present potential risks. In the wrong hands, malicious actors could use AI agents to compose phishing emails by matching leaked data. These can also be used to create fake profiles for scams and scour online data to identify targets for romance scams.
AI governance and regional frameworks
The responsible deployment of AI agents requires careful consideration of ethical and regulatory frameworks. Irecki highlights that "various countries in the Asia Pacific, including Singapore, have launched AI frameworks, such as Singapore's National AI Strategy."
These frameworks emphasise "accountability, fairness, data minimisation, security, transparency, monitoring, and auditing, helping businesses understand the best practices for adopting and governing AI responsibly."
He anticipates that "AI governance platforms will become more common" in 2025, providing a centralised means of managing policies and ensuring "transparent and responsible usage" across multiple AI agents.
The future of AI agents
AI agents will evolve and become more integrated into the workforce. OpenAI's Sam Altman believes that "in 2025, we may see the first AI agents' join the workforce' and materially change companies' output".
McKinsey research estimates that generative AI could add between US$2.6 trillion and US$4.4 trillion annually to the global economy.
What's next?
No one argues that the hype surrounding AI agents is substantial. Therefore, it is crucial to approach the technology with a realistic understanding of its current capabilities and limitations. As AI technology advances, AI agents will eventually become more reliable, versatile, and integrated into the workforce, but it is essential to address the challenges and potential risks associated with their use.
For Irecki, collaboration across multiple disciplines and divisions will be crucial when integrating AI agents into processes. This requires a holistic approach to ensure all aspects, such as data quality, governance, and security, are addressed.
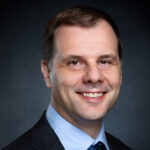
"Bringing in the right partners is key, as different tools and expertise for data quality, liquidity, governance, and security tools from other providers are needed to support the transformation." David Irecki
Click on the PodChats and listen to Irecki share his perspective on the realities of AI agents – what you and I need to know, separating hype from reality.
- Define AI agents as understood by most Asian users (both tech and non-tech).
- What key factors differentiate AI agent hype from reality for organisations?
- Since you brought it up, how can businesses ensure high data quality for effective AI deployment?
- Scale-out issues were a recurring topic when RPAs came out, and they may be part of the challenge with AI. From your experience, what common pitfalls should organisations avoid when adopting AI agents?
- One of AI's promises is improving productivity—at least, this is the hope. In what ways can AI agents drive productivity and cost savings in specific industries? Are there any pitfalls to avoid?
- How can AI agents enhance customer experiences across different sectors?
- How can businesses maintain accountability and governance while leveraging AI agents effectively?
- This 2025, what is your advice for lines of business, finance, IT, and security to work together to design, architect or transform processes so they can avail of the promise of AI agents without introducing unnecessary risks in the process?