In 2025, the landscape of technology adoption is transforming rapidly, with AI and automation at the forefront of this evolution. As organisations strive for greater efficiency and innovation, the roles under the Chief Information Officer (CIO) have become pivotal in navigating this shift.
The AI market is evolving toward monetisation and widespread adoption, focusing on resilience, workforce transformation, and managing regulatory changes. Priorities are being driven toward ethical AI integration, explainability, and advancing modernisation as organisations aim for responsible, compliant, and future-ready AI implementation.
IDC predicts that Asia/Pacific business leaders will demand an 80% success rate of generative AI (GenAI) adoption by 2027. This is an impressive prediction given that FutureCIO polls reveals that identifying use cases at the working level remains the biggest hurdle among technology leaders attending the FutureCIO Hong Kong conference in October 2024.
"The adoption of AI and GenAI in the APJ region is driving a notable shift in business strategies and value creation. Companies are leveraging AI to boost efficiency and enrich customer experiences, opening doors to fresh growth opportunities," says Vinayaka Venkatesh, senior market analyst, Data & Analytics, IDC Asia/Pacific.
Imagine you are the CEO of a Fortune 100 company. You are investing in exciting technologies like GenAI to grow your business. But, like many leaders, you are facing a storm of challenges: interconnected applications, wasted cloud spending (27% on average!), and a shortage of skilled IT talent.
According to a HBR study, 82% of enterprise leaders say IT complexity impedes success! All this complexity creates silos, outages, and wasted resources, hindering seamless integration and modern service adoption.
At the IBM Tech Innovation Summit in Thailand, putting artificial intelligence (AI) in action will be the theme of the event.
How should leaders in Asia, including CIOs, navigate the opportunities and challenges that come with the adoption of AI and GenAI in the workplace? Kalyan Madala, IBM's APAC Field CTO, uncovers enterprise AI values from +AI to AI+ in the Asian Century.
Architecture
Leading a technical team across APAC at IBM, Madala observed great interest in AI in the region: "Customers are learning what works, what doesn't, and what it takes to make AI effective. They are assessing the return on investment, weighing costs, risks, and opportunities."
While AI adoption accelerates in APAC, enterprises face significant architectural challenges in integrating AI and automation into existing enterprise systems. Madala noted that selecting suitable AI models and managing risks are among the most pressing concerns enterprises in the region face. While these are common challenges globally, the APAC region presents its own unique set of hurdles.
AI adoption in the region is shaped not only by technical constraints but also by language diversity. With over 20 countries and over 3,000 languages, including Mandarin, Hindi, Bangla, Indonesian, Japanese, Filipino, Malay, Vietnamese, and Tamil, the region's diversity adds a layer of complexity to AI implementation. Madala pointed out that understanding linguistic nuances poses significant challenges even for basic large language models (LLMs).
Moreover, with the regulatory landscape in APAC still evolving and the pace varying from country to country, Madala believes it will be a significant challenge for enterprises to navigate. He says the regulatory focus has expanded from data residency to AI-specific guidelines and recommendations.
Beyond compliance concerns, AI's rapid expansion is also reshaping the workforce, raising concerns about job displacement, which is exacerbated in some countries in APAC compared to others. A report from the Asian Development Bank noted that the expansion of generative AI and large-language models affected higher cognitive and nonroutine content.
"Jobs that require nuanced judgment, creative problem-solving, or intricate data interpretation—traditionally the domain of highly skilled professionals—can now be augmented or even replaced by advanced AI algorithms, potentially exacerbating inequality across and within occupations," it highlighted.
The IBM executive noted that as AI brings numerous challenges to the architectural design process, leveraging AI tools for automation and augmentation can enhance productivity across various business processes.
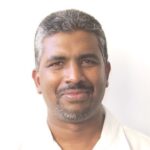
Madala urges organisations to identify the risks in each process to minimise them as they become more dependent on AI. He also highlighted the importance of Governance, Risk, and Compliance (GRC) practices in identifying and mitigating risks associated with AI adoption in their workflows. Madala outlined a structured approach to risk management—identifying threats, testing solutions, and applying controls.
Development and engineering
While enterprises struggle with architectural hurdles, another layer of complexity emerges in software development, where AI is reshaping engineering workflows. AI is no longer just a tool for assisting developers; it is increasingly automating coding, testing, and deployment. This raises questions about the role of a developer—are they writing code or instructing an AI to do so?
For Madala, the application development lifecycle is evolving, creating new responsibilities and challenges for developers. He said they need to be more careful, conscious, and deliberate in selecting the appropriate tools for automation.
He cautions organisations about "black boxes," or AI models that lack transparency and possibly introduce unknown risks. Madala urges organisations to adopt explainable AI and continuously monitor AI models to ensure the reliability and security of output and clean supply chains.
"If the supply chain is clean, how the model was trained is clean, and if they can understand how the model behaves, I can have more certainty and more comfort about the output," he said. "I think, in a sense, you are rewiring how we approach development, identifying risks, and mitigating them."
According to Madala, adopting AI necessitates a mindset shift: "I think, in a sense, you are rewiring how we approach development, identifying risks, and mitigating them. I would say we need to rethink the entire value chain. We need to change our mindset and approach to it."
While acknowledging AI's potential to improve productivity and optimise resource use, he said it is crucial to identify and mitigate risks proactively.
Madala also warned against overreliance on technology: "We can't let complacency come into the value chain when you're deploying, creating, and deploying critical applications." He advises developers not to "be lulled into a false sense of comfort and complacency."
Operations and reliability
With AI changing how software is built, it's only natural that its impact extends to operational reliability and system performance. Madala posits that modern architecture is evolving towards abstraction and declarative approaches, further complicating application deployment.
He said applying AI to operations enables faster problem detection, root cause analysis (RCA), and the potential for automated mitigated actions. However, like a double-edged sword, Madala foresees operational challenges in maintaining system reliability as organisations adopt AI-driven automation tools.
As quality improves, our expectation of quality also increases. He emphasised that poor data quality hinders AI's ability to generate reliable insights:
"As quality improves, our expectation of quality also increases. What was considered a high-quality dataset 20 years ago no longer meets today's standards. We want far richer data sets, more integrated data sets, and more insights than we have, which will continue to rise as we go." Kalyan Madala
Madala also posits that traditional data analysis relies on predefined queries, where analysts select datasets, apply specific filters, summarise and group data, and interpret insights based on predefined expectations. On the other hand, AI enables a more exploratory approach, where the system autonomously finds correlations that humans might not have considered. He posits that organisations can leverage AI's ability to generate unexpected insights when they retrain their approach.
As AI assumes greater responsibility, organisations must prioritise explainability, risk management, governance, and bias detection.
To address the risk of biased outputs, companies like IBM have developed tools such as AI 360 to detect bias in data sets and machine learning models and mechanisms.
"It's key that we look out for these biases and help ensure that we build fair AI systems," Madala said.
From +AI to AI+
The increasing reliance on AI in business operations signals a shift: AI is evolving from a supporting role (+AI) to becoming the foundation of innovation (AI+).
As the region grows from adopting AI to leading in AI-driven transformation, tech leaders must redefine their roles, mitigate risks, and combat complacency to ensure long-term success.
Click on the link to know more about the IBM Tech Innovation Summit in Thailand and how you can harness the potential of AI for your organisation.
Click on the PodChats and listen to Madala to uncover enterprise AI values
1. Architecture
- Opportunities: How can we leverage AI-driven tools to enhance our architectural design processes, and what specific benefits can we expect in terms of efficiency and scalability?
- Challenges: What are the key architectural challenges we face in integrating AI and automation into our existing enterprise systems, and how can we overcome them?
- Risks: With the increasing reliance on AI in architecture, what potential risks do we face regarding data privacy and compliance, and how can we mitigate these risks?
2. Development & Engineering
- Opportunities: In what ways can AI enhance our coding and testing processes, and how can we measure the return on investment from these advancements?
- Challenges: What hurdles do our development teams encounter when implementing AI and automation in the software development lifecycle, and how can we address them?
- Risks: As we automate more development tasks, how do we ensure that quality and security are not compromised, particularly with the rise of AI-generated code?
3. Operations & Reliability
- Opportunities: How can AI enhance our incident response and system monitoring capabilities, and what specific tools should we consider in our strategy?
- Challenges: What operational challenges do we foresee in maintaining system reliability as we adopt AI-driven automation tools, and how can we prepare for them?
- Risks: What are the potential risks of over-reliance on AI for operational decisions, and how can we ensure human oversight remains effective?
4. Data & Analytics
- Opportunities: How can we leverage AI to uncover new insights from our data that were previously unattainable, and what processes should we put in place to act onthese insights?
- Challenges: What are the key challenges in ensuring data quality and governance as we implement AI-driven analytics tools, and how can we address them?
- Risks: What risks do we face regarding data bias in AI models, and how can we implement strategies to ensure fairness and transparency in our analytics processes?