As more artificial intelligence (AI) and large language models pop up in the market, including open source alternatives, organisations will have to do due diligence in assessing the different options to identify the right fit for their use cases.
With AI, the mandate last year for most businesses was simply to do something involving the technology. The focus now has moved to returns on investment (ROI) and rolling out AI at scale, said Mike Mason, chief AI officer at tech consultancy Thoughtworks.
Organisations want to move AI beyond experimentation and they need to do so with testing and evaluations, so they can ensure they are using AI responsibly, Mason said in a phone interview with FutureCIO.
He pointed to the emergence of new reasoning models in the past year, which he described as significant since these models can power more useful work on the behalf of humans. China’s DeepSeek, for example, is a reasoning model, he noted.
These AI models can decide autonomously how much time to spend on a question and allocate more time on a complex query that requires deeper research, he said, referring to the “slow AI” approach.
Slow AI is often described as a concept that focuses on human-centric design and ethical reasoning, with such tools built to augment human creativity and capabilities. It contrasts with the traditional “fast AI” concept, which seeks to operate and generate results quickly, relying on patterns to make decisions.
It opens up more options for enterprises to explore which systems they can use to run their AI models, including open-weight large language models (LLMs), Mason said.
In open-weight models, pre-trained parameters or core settings in the model, called weights, are made publicly available, while other key components such as the training code, datasets, and methodology are not.
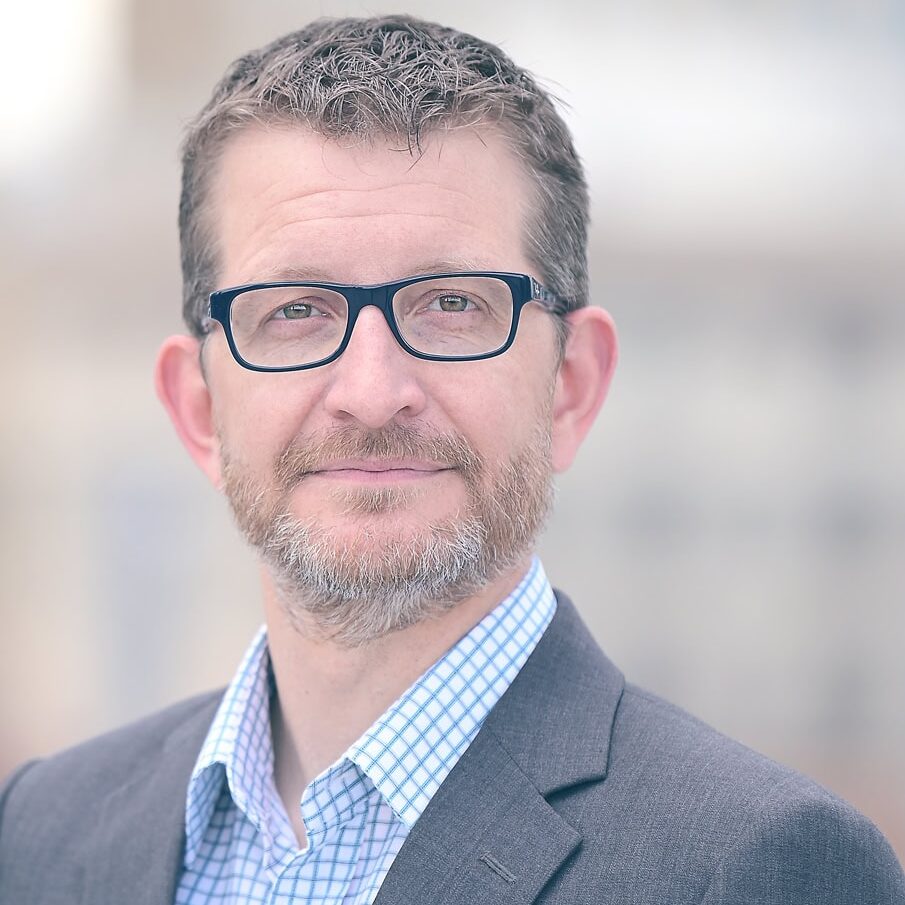
Described as a balance between open source and proprietary frameworks, open-weight AI models can be licensed under certain terms that permit broader use and reuse. Examples of open-weight models currently include LlaMa2 and Mistral 7B-Instruct.
OpenAI CEO Sam Atman earlier this week posted on X that the AI company was planning to release its first open-weight language model with reasoning capabilities.
Do not adopt AI models blindly
It allows for enterprises to run AI models on a cloud platform as well as infrastructure they can control. It also highlights the need for them to assess the merits of different AI models, whether open source or otherwise, based on their own use case.
Companies have to go further than just benchmarking and look at evaluations, or evals, to assess beyond “raw performance”, Mason said.
They need to look at how the AI model behaves when responding to the same question that is asked in different ways or when asked the same question multiple times. If a different answer is generated each time, the organisation then needs to look deeper into how the AI model arrives at its decisions, he explained.
This should include assessing LLMs for potential bias in its training and output.
“Organisations cannot afford to adopt AI models blindly,” Mason said, stressing the importance of evaluating these platforms for reliability, security, and fairness.
He noted that traditional benchmarks such as SOTA listings can be useful, but do not always indicate real-world performance.
SOTA, or state of the art, benchmarks rank best-performing models or algorithms in a specific segment, such as AI modelling or techniques.
Mason suggested that organisations conducted in-depth evaluations to assess AI behaviour in their specific use cases. He pointed to techniques, such as input sensitivity testing, hallucination detection, and neural activation analysis, which he said were emerging as useful tools to better understand AI model decision making.
Potential bias in AI models should be addressed as it is a key concern especially in Asia, where organisations may operate in environments that are unique to the region, he said.
He added that there likely will be a rise in “sovereign AI” where nations work to have more control on AI models.
According to World Economic Forum (WEF), sovereign AI aims to bolster a nation’s ability to “protect and advance” its interests through the use of AI, building their own AI algorithms to achieve “strategic resilience”.
Over time, it also will enable countries to reduce reliance on foreign AI technologies, by developing their own AI capabilities and ensuring access to critical data and technologies locally, said WEF.
According to Mason, Thoughtworks is working with AI Singapore on a research project to build on the government agency’s Southeast Asia-focused LLM, SEA-LION version 3.
SEA-LION, or Southeast Asian Languages in One Network, is an open source LLM touted to better represent the region’s diverse population mix and understand contextual nuances in cultures and languages. The latest version is built on 200 billion Southeast Asian tokens from 11 official regional languages and touted to run on custom post-training with instruction tuning.
Apart from Thoughtworks, AI Singapore works with various industry partners on building out SEA-LION, including Google, Nvidia, Alibaba.com, and Singtel.
Mason said: “Our focus is on developing the world’s first language embedding model to tackle the unique challenges of multilingual AI applications in Southeast Asia. This work is especially relevant for enterprises looking to deploy AI solutions in languages beyond English and Chinese, where existing models often struggle with.”
To open source or not to open source
Thoughtworks says it uses and contributes to open source software wherever possible, but Mason noted that organisations should weigh the benefits and challenges of doing so in their own environment.
Asked how businesses should assess whether they should opt for an open source or proprietary AI model, he said there is no one-size-fits-all solution.
Open-weight or open source models provide transparency and flexibility, while closed models may offer stronger security and technical support, Mason said.
“Enterprises need to weigh the trade-offs carefully, particularly in relations to compliance, data security, and operational risks,” he noted.
He added that there were many AI risks, such as bias, data leaks, and unpredictable outputs, but these are foreseeable and can be managed with the right mitigation strategies.
Organisations also should be looking at such issues and ensuring that these are addressed from the start, he said.
“Security can’t be an afterthought; it needs to be embedded in the AI development lifecycle from day one,” he noted.