In recent years, the industry has witnessed the migration of machine learning (ML) closer to the data source to create a better user experience and enhance privacy. To ease the challenges in design and development and accelerate adoption, many companies are offering development platforms, tools, libraries, and solutions for edge ML applications. As the adoption of these edge ML enablement platforms and solutions continue to grow, ABI Research is forecasting the edge ML enablement market to exceed US$5 billion by 2027.
Edge machine learning brings a significant upgrade in user experience, privacy protection, and cost optimization for end users. Popular applications such as image recognition, predictive maintenance, and speech processing augment human experts with data-driven insights. However, to create a production-ready, enterprise-grade, edge ML solution, enterprises need to invest in large amounts of skill sets.
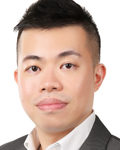
“To truly benefit from the benefits of edge ML applications, enterprises need to make sure their edge ML applications are accurate, optimised, and closely monitored to prevent biases and model drift. This means enterprises must build a team of data scientists, device and embedded engineers, and project managers, which can become very expensive very quickly,” explains Lian Jye Su, research director at ABI Research.
He added that edge ML enablement vendors offer enterprises a hand in their Artificial Intelligence (AI) journey. They create user-friendly tools and libraries to lower the barrier of adoption.
Specifically, edge ML enablement vendors enable enterprises to manage and govern their data for ML training and inference, select the suitable models, train and test the model, create an inference engine, and deploy and monitor the ML through edge ML Operations (MLOps). Many are offering application-specific solutions for dedicated applications such as audio classification, predictive maintenance, gesture recognition, and material detection. ABI Research has identified four major categories of edge ML enablement vendors, namely public cloud vendors, edge ML silicon vendors, platform-focused vendors, and technology-focused vendors.
Platform-focused vendors like Edge Impulse, Imagimob, and SensiML offer the most comprehensive databases, tools, libraries, and solutions for edge ML applications. Nonetheless, the rest of the field has unique value propositions. Technology-focused vendors like Latent AI and Plumerai focus on specific technology within the data science and ML stack. Edge ML silicon vendors like DeGirum and NXP offer developers highly optimized full-stack hardware and software solutions. Lastly, public cloud vendors allow enterprises to leverage the flexibility and scalable of cloud infrastructure when developing their edge ML applications.
“The market is still at a nascent stage. Most edge ML projects still fail to move past the experimental phase. Edge ML enablement vendors must continue to improve their low-code or no-code user experience for non-AI experts, expand edge ML hardware support, provide application-specific solutions and services, and ensure a high level of ML explainability to ensure legal compliance,” concludes Su.