Generative AI (GenAI) is transforming more than half (51%) of business in the Asia-Pacific region, from speeding up app development to revolutionising customer interactions. Yet, while 59% of Singaporean businesses have embraced GenAI, only a fraction of projects move beyond the proof-of- concept stage. In fact, analysts predict that by 2025, 30% of GenAI projects globally will be abandoned after their initial pilots.
This raises questions for business leaders planning on going big in GenAI adoption: Why are these businesses struggling to scale GenAI, and how can they tackle successful deployment in 2025?
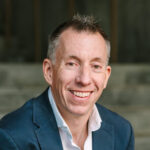
Fuzzy goals, scarce talent
The trouble starts at the top. The push for GenAI is often a top-down attempt from business leaders to churn out an AI story to showcase their capabilities and establish a foothold in the space.
However, when these mandates filter down to the Chief Technology Officer or IT innovation lead, it lacks clarity and alignment on the specific problems that AI is meant to address. Whether it’s as straightforward as implementing a chatbot for customer support or tackling more complex solutions, the goals are often ambiguous, leading to uncertainty around project execution.
Compounding the issue is the severe shortage of skilled talent. Despite Singapore’s ambitions to become a global AI hub, 75% of professionals in the finance and technology sectors report difficulty finding qualified AI talent, particularly for middle and senior management roles. Without the right expertise, such initiatives will stall, limiting the ability to scale advanced AI solutions.
The volatile ground of GenAI integration
Beyond organisational challenges, the GenAI landscape itself changes daily. The rapid evolution of large language models (LLMs) poses a moving target for enterprises, and the fast-paced cycle creates instability, as frequent updates lead to compatibility issues with legacy systems and outdated implementations.
For instance, 43% of Singaporean businesses report that their data and systems are not effectively interconnected, requiring employees to toggle between dozens of applications. Such fragmented systems make it harder to integrate new GenAI tools, driving up costs and complexity.
The performance of AI models in the market right now further complicates matters. While GenAI models may excel in controlled tests, they can behave inconsistently in real-world scenarios. For example, a chatbot trained to provide customer support might struggle to deliver reliable answers when dealing with nuanced queries, eroding trust in the technology.
Minimising GenAI variability with GraphRAG
Despite the challenges of GenAI deployment, businesses can overcome these hurdles by adopting innovative approaches like GraphRAG (Graph-based Retrieval Augmented Generation). This method integrates GenAI models with a knowledge graph - a structured representation of data that maps the relationships between various data points, much like a digital mind map.
Imagine a network of interconnected data points (e.g., a product feature, a customer inquiry, or a policy). Knowledge graphs highlight how these points are related, enabling GenAI outputs rooted in factual, context-rich information, enhancing accuracy and reliability.
To successfully implement GraphRAG, businesses must begin by defining clear use cases where GenAI can create measurable value. These might include areas like fraud detection, recommendation engines, or supply chain optimisation.
A robust and skilled technical team is also essential and organisations should invest in cross- functional training, particularly in graph-specific query languages, and establish processes for continuous performance monitoring. Such a team ensures that the knowledge graph operates efficiently, even as the underlying dataset grows in complexity.
Additionally, leaders must foster a culture of iterative improvement so that teams regularly refine and experiment with the knowledge graph to unlock new insights and enhance performance over time.
As GenAI reshapes industries, businesses face both significant opportunities and pressing challenges.
To be successful in GenAI adoption, business leaders need a combination of clear objectives, skilled teams, and advanced technologies like GraphRAG to overcome variability and integrate GenAI into scalable, stable workflows.
By addressing these factors, organisations can transform today’s pilot projects into tomorrow’s transformative solutions, positioning themselves at the forefront of the AI revolution.