Recently, I had the honor of presenting Forrester’s predictions for 2024 to over a thousand tech and business leaders. There were, unsurprisingly, a ton of questions about generative AI (genAI). I’d like to answer some of the more technical questions that we received. My colleagues Sharyn Leaver and Laura Ramos wrote a great post rounding up some the top questions about leveraging genAI in a business context.
For even more insight into how tech leaders can leverage genAI in 2024, check out our on-demand Predictions 2024 webinar specifically geared toward tech leaders.
Building a genAI strategy
Here are five steps you can take to launch your genAI strategy:
- Engage with employees to build a safe and flexible set of guidelines around unofficial genAI tools.
- Explore initial genAI use cases. Include both internally developed capabilities and vendor-supported functionalities. Empower one team to coordinate and catalog these use cases.
- Center initial deployments around internal use cases that will enhance employee productivity, research, content drafting, or customer engagement and support.
- Begin preparing enterprise data, both for use by genAI applications and to be leveraged as instruction-tuning data sets. This should start with unstructured data that can either be accessed by traditional keyword or lexical indexing or by embeddings in a vector database.
- Work from the top down and bottom up to link employee enthusiasm, executive interest, and adjacent applications for AI and genAI. Deliberately work against shadow AI.
Making data AI-ready
Companies should hone their basic data management and governance practices. Currently, genAI relies mostly on unstructured data to ground its outputs, so start by getting key unstructured data sets managed, indexed for search, and enriched with metadata to support the retrieval and delivery of the data to large language models (LLMs). Making sure that you can access the needed data sources (with the proper enterprise permissions) will enable the consumption of this information by genAI tools.
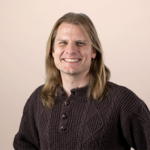
GenAI in data engineering or data management
Data engineering and data management currently have a more limited set of use cases in the genAI space than other applications, but there is significant potential. Some companies have started to leverage LLMs to create metadata from unstructured content, such as call center transcripts — extracting topics, entities, themes, etc. There are also early applications using LLMs to assist in creating and maintaining data dictionaries. The LLMs can help draft data definitions after being fed schemas and relationships. Additionally, there are a few vendors who are using LLMs to generate synthetic data for use cases including model training, product demonstration, and application development and testing. These are mostly on the unstructured side today, with a few doing structured data, as well.
Monitoring and evaluating AI projects
The role of risk management includes identifying genAI use cases with the biggest potential upside for the organization. In our research on the transformational chief risk officer (CRO), Forrester describes a fundamental shift in risk management from a transactional function focused on reducing costs to a transformational one that enables organizations to capitalize on new opportunities. The transformational CRO helps their organization make better, faster strategic decisions while avoiding unnecessary or costly missteps.
This new focus puts risk management in the driver’s seat to identify the areas and use cases where genAI can add the greatest value quickly. This includes how to apply genAI ethically and which AI projects will require additional guardrails, oversight, and controls. By focusing on outcomes, risk management can measure genAI’s impact to customer experience, employee experience, quality, safety, and innovation, rather than simply the financial impact from a risk event or compliance violation. Risk management will provide the air cover to realize the ROI of genAI.
Originally posted by Rowan Curran on Forrester