Arguably a byproduct of the increased use of, or intent to use, generative AI (GenAI), synthetic data addresses the data shortfall needed for training AI algorithms while enhancing security and privacy. It allows organisations to avoid collecting sensitive information, thus ensuring compliance with stringent privacy regulations.
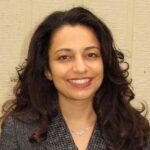
This is particularly crucial in sectors like healthcare and finance, where data protection is paramount. Rena Bhattacharyya, chief analyst and practice lead for Enterprise Technology and Services at GlobalData, comments that utilising synthetic data, allows firms to conduct risk evaluations, fraud prevention, and predictive analytics without exposing real user data.
This reduces the risk of data breaches and enhances operational efficiency, making synthetic data a secure alternative for various applications across industries.
Synthetic data risks
But as history tells us, new technologies often come with new risks that have yet to be discovered.
Zeid Khater, analyst at Forrester, suggests one such risk might arise from misrepresentation. This happens when attempting to up-sample for a missing attribute or element in the data, that may be missing for some real-world reason. “Simply augmenting a missing element (like a demographic group) might bias your sample or undermine the factors that initially led to the absence of a particular group in your dataset to begin with,” he explains.
He also posits dimensionality as potentially posing a problem. “For high dimensional data, particularly for structured data, there can be accuracy and reliability issues often associated with what data scientists refer to as “the curse of dimensionality,” he continues.
Role of synthetic data in AI model training
While academia has been using it for nearly 30 years, synthetic data has only entered mainstream commercial use in recent years.
According to Khater, the idea to generate synthetic data as a tool for broadening access to sensitive microdata was proposed for the first time three decades ago. “While first applications of the idea emerged around the turn of the century, the approach gained momentum over the last ten years, stimulated at least in parts by some recent developments in computer science,” he continues.
Gartner estimates that as of 2021, only 1% of data was synthetic, but that by 2024 that figure will astonishingly grow to 60%.
Khater says when used in combination with small high-quality real data, synthetic data has proven to produce higher performant models (see Microsoft’s research on their use of synthetic data to train their phi-model: [2306.11644] Textbooks Are All You Need (arxiv.org).
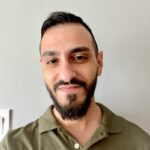
He also believes that synthetic data will continue to be used for training data for prompt engineering and retrieval augmented generation (RAG) testing to ensure the outputs are working as designed, for things like driver-monitoring systems (images of drivers falling asleep at the wheel to alert drowsy or sleepy drivers), digital twins, simulation testing and more.
“The ease of generating synthetic data via GenAI (GANs and VAEs) has made it increasingly more popular to rely on synthetic data for these use cases and similar ones.” Zeid Khater
Approaches to synthetic data generation
While users can always build their synthetic data, Khater reckons organisations will face multiple challenges related to dimensionality.
“There are many new data and platforms that act as standalone vendors including Gretel, Tonic, DataCebo, Franz, MostlyAI, and Mockaroo. These provide either the data itself or a platform to integrate synthetic data creation and usage inside your existing tech stack,” lists out Khater.
He also points to others who specialise in leveraging the data along with LLMs to generate dynamic market research augmentation and product development such as DayOne Strategy and Synthetic Human/Fantasy AI (AI Synthetic Humans: Revolutionising User Research & Team Collaboration | Fantasy Interactive (synthetic-humans.ai). “You may also find that your existing Customer Analytics service providers can do this for you as well such as Fractal or Tredence,” suggests Khater.
Use synthetic data while staying compliant
Asked how can organisations leverage synthetic data while complying with data privacy regulations, Khater argues that synthetic data is in itself compliant because in most cases it can’t be traced back to the original from which it was synthesised (See Gretel’s Differential Privacy).
However, he cautions that in some cases, even an original from which to create synthetic data is problematic due to several reasons: it either doesn’t exist (rare diseases) or is highly regulated (financial or patient data).
“Some data scientists have gotten around this by manually building smaller datasets of roughly 200 rows or so, then have them validated by subject matter experts or others with access to the original data to confirm statistical accuracy and augment off of that,” continues Khater. For example, MedWGAN-based synthetic dataset generation for Uveitis pathology – ScienceDirect.
For best results
Given the growing roster of vendors offering solutions, enterprises will need to identify which GenAI techniques will provide the best results for specific data needs. How should organisations evaluate the effectiveness of such approaches?
Khater says this starting point is business intent. He notes that GANs (generative adversarial networks) often generate the most realistic data. He warns that there is little control over the resultant dataset. “On the other hand, VAEs (variational autoencoders) provide some control through the manipulation of “latent space” – in simple terms, a compressed version of the original dataset that holds all its essential dimensions – but tends to be less accurate than GANs,” he comments.
“Your business intent and use case will determine which method makes the most sense based on those criteria. In some instances, rules-based synthetic data might still be leveraged for maximum control, though it is usually too simplistic and therefore not useful for complex data relationships,” he elaborates.
Measuring the impact
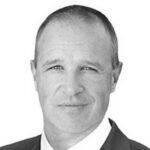
Alexander Linden, VP analyst at Gartner, says synthetic data makes AI possible where lack of data makes AI unusable due to bias or inability to recognise rare or unprecedented scenarios.
“Real-world data is happenstance and does not contain all permutations of conditions or events possible in the real world. Synthetic data can counter this by generating data at the edges, or for conditions not yet seen,” he explains.
When measuring the impact of synthetic data on AI initiatives will require looking at, Khater suggests looking at speed to value. “Access to data might typically slow down data for insights if stringently governed in an organisation or in instances where there is no data, low-quality data, or not enough data,” he elaborates.
He also suggests measuring speed along with compliance. “You can move faster without the fear of regulatory backlash. And of course, model performance via benchmarking,” he concludes.